Cristina González
B.Sc. Computer Science
B.Sc. Biomedical Engineering
Universidad de los Andes
I am a Ph.D. Candidate in Engineering and a researcher at the Center for Research and Formation in Artificial Intelligence (CINFONIA) supervised by Prof. Pablo Arbeláez at Universidad de Los Andes in Bogotá, Colombia. My main interests include visual recognition, biomedical image analysis, egocentric vision and vision & natural language processing. I am participating in Ego4D!
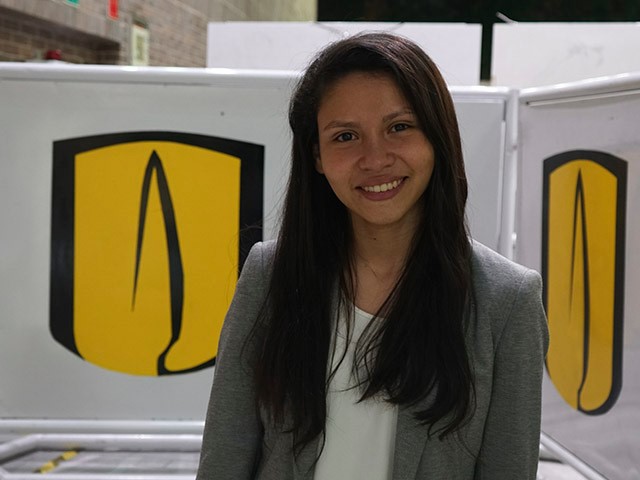
Publications
Ego4D: Around the World in 3,000 Hours of Egocentric Video
Kristen Grauman et al.
CVPR, 2022 (Oral presentation).
Project pagePanoptic Narrative Grounding
Cristina González, Nicolás Ayobi, Isabela Hernández, José Hernández, Jordi Pont-Tuset and Pablo Arbeláez
ICCV, 2021 (Oral presentation).
Project pageComparative validation of multi-instance instrument segmentation in endoscopy: results of the ROBUST-MIS 2019 challenge
Tobias Roß et. al.
Medial Image Analysis, 2021.
PharmaNet: Pharmaceutical discovery with deep recurrent neural networks
Paola Ruiz, Natalia Valderrama, Cristina González, Laura Daza, Carolina Muñoz-Camargo, Juan Carlos Cruz, and Pablo Arbeláez
PLOS ONE, 2021.
Project pageSIMBA: Specific Identity Markers for Bone Age Assessment
Cristina González*, Maria Escobar*, Felipe Torres, Laura Daza, Gustavo Triana and Pablo Arbeláez
MICCAI, 2020.
Project pageISINet: An Instance-Based Approach for Surgical Instrument Segmentation
Cristina González*, Laura Bravo* and Pablo Arbeláez
MICCAI, 2020.
Project pageHand Pose Estimation for Pediatric Bone Age Assessment
Maria Escobar*, Cristina González*, Felipe Torres Figueroa, Laura Daza, Gustavo Triana and Pablo Arbeláez
MICCAI, 2019 (Oral presentation).
Project pageAn Empirical Study on Global Bone Age Assessment
Felipe Torres, Cristina González, Maria Escobar, Laura Daza, Gustavo Triana and Pablo Arbeláez
SIPAIM, 2019.
Project pageExperience
Ph.D Research Assistant, Center for Research and Formation in Artificial Intelligence (CINFONIA)
Universidad de los Andes, Bogotá
- Member of the Biomedical Computer Vision (BCV) group at Uniandes led by Prof. Pablo Arbeláez.
- Applying computer science skills in biomedical imaging problems.
- Working on teams to develop automated solutions to biomedical problems.
- Writing paper submissions for international conferences.
Undergraduate Research Assistant, Biomedical Computer Vision
Universidad de los Andes, Bogotá
- Member of the Biomedical Computer Vision (BCV) group at Uniandes led by Prof. Pablo Arbeláez.
- Applying computer science skills in biomedical imaging problems.
- Working on teams to develop automated solutions to biomedical problems.
- Writing paper submissions for international conferences.
Deep Learning Research Intern
Adobe Research
- Intern at the Deep Learning Lab advised by Fabian Caba.
- Applying deep learning algorithms in video analysis problems.
- Working on teams to develop automated solutions to video analysis problems.
Undergraduate teaching assistant
Universidad de los Andes, Bogotá
In carge of assisting students during midterms and office hours, designing and grading midterms, designing review exercises for each class and review lessons.
- Analysis and Processing of Biomedical Images
- Scientific Programming
- Algorithm and Object-Oriented Programming I
- Freshman students' Support Program
Awards
Adobe Research Women-in-Technology Scholarship 2020
Adobe Research
Recognize outstanding undergraduate female students anywhere in the world who are studying computer science
Robust Medical Instrument Segmentation (ROBUST-MIS) Challenge 2019
Laura Bravo*, Cristina González* and Pablo Arbeláez
Endoscopic Vision Challenge, MICCAI 2019
1st place:
- Multiple Instance Detection task.
- Normalized Surface Dice Generalization ranking of the Multiple Instance Segmentation task.
2nd place:
- Dice Similarity Coefficient Generalization ranking of the Multiple Instance Segmentation task.
- Dice Similarity Coefficient Robustness ranking of the Multiple Instance Segmentation task.
- Normalized Surface Dice Robustness ranking of the Multiple Instance Segmentation task.
Our performance shows that our method was the most robust of the competition.